Radiology plays a major role in the diagnosis and treatment of various diseases. Deep-learning, also known as hierarchical learning, is a type of machine learning involving algorithms and based on learning data representations. Deep-learning is used in the field of medicine, particularly in radiology.

What is Deep-Learning?
Deep-learning is a type of machine learning method that helps machines and computers learn by example. In deep-learning, the machine or computer learns about classification tasks directly from sound, text, or image input.
Programs using deep-learning can perform tasks accurately and often at a higher level than humans. For example, zoloft and pain killer deep-learning is the key technology of self-driving cars that allows lets them to detect pedestrians or stop signs. Simply put, deep-learning is a form of artificial intelligence.
Deep-learning is a branch of artificial intelligence in which networks of basic interrelated units are used to recognize patterns in data to solve complicated problems. Deep-learning has been used in several sophisticated tasks related to medical imaging.
Deep-Learning in Radiology
Importance of Radiology to Medical Practice
Medical imaging is an important diagnostic and treatment tool for many human diseases. In diagnostic imaging, a series of tests are used to capture images of various body parts. These tests provide physicians with images that can be used to detect abnormalities in body organs.
Many imaging modalities are used to view internal body structures. Examples include X-rays, computed tomography scans, magnetic resonance imaging, and positron emission tomography.
Imaging studies are especially important in cancer detection and diagnosis. Early identification of cancer and other diseases allows for earlier treatment, which may improve patient outcomes.
How Deep-Learning is Used in Diagnostic Radiology
Deep-learning is an important tool used in radiology and medical imaging. Deep-learning techniques can be used to precisely segment organs based on their boundaries to provide accurate measurements. Furthermore, deep-learning techniques can be applied to the detection of tumor growth and to discriminate between benign and malignant structures.
Although deep-learning requires more data than traditional machine learning, it is also easier to use. Because most deep-learning systems use neural network designs, these models are often referred to as deep neural networks. The word “deep” refers to its multi-layered structure.
In a conventional neural network, there are only 2 or 3 hidden layers. In contrast, deep networks may have hundreds of layers. As a result, deep-learning models can be used to analyze and use large sets of neural network architectures and data to provide more accurate representations for imaging studies.
Traditionally, deep-learning has been applied to single images. However, the use of deep-learning in radiology is more complex because computed tomography and magnetic resonance imaging produce thousands of images. Thus, deep-learning models require more complex computational algorithms in the field of radiology. Deep-learning is now being used in clinical radiology to detect, quantify, and segment lesions and to diagnose and classify disease.
Conclusion
Deep-learning has dramatically improved the performance of computer algorithms in medical practice. Medical technology has evolved to allow the extraction of crucial information from images that may have been more difficult to interpret using traditional methods. To date, deep-learning methods have been used in breast and lung cancer screening and brain segmentation studies.
Research on deep-learning technology has shown promising results that can be applied within medical practice and for other uses. In the future, deep-learning is expected to further enhance diagnosis and treatment of various diseases.
Sources
- https://arxiv.org/abs/1802.08717
- https://www.ncbi.nlm.nih.gov/pubmed/29606338
- https://www.sciencedirect.com/science/article/pii/S1076633218301041
- https://www.sciencedirect.com/science/article/pii/S1546144017316721
Further Reading
- All Artificial Intelligence Content
- Artificial Intelligence in Cardiology
- Artificial Intelligence and Deep Learning in Medicine
- Machine Learning in Drug Development
- Artificial Intelligence and Life Science
Last Updated: Oct 24, 2018
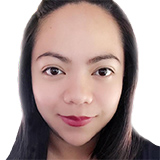
Written by
Angela Betsaida B. Laguipo
Angela is a nurse by profession and a writer by heart. She graduated with honors (Cum Laude) for her Bachelor of Nursing degree at the University of Baguio, Philippines. She is currently completing her Master's Degree where she specialized in Maternal and Child Nursing and worked as a clinical instructor and educator in the School of Nursing at the University of Baguio.
Source: Read Full Article