In a recent study published in Nature Biomedical Engineering, researchers proposed the utilization of a vision transformer model to decode surgeon activities from surgical videos.
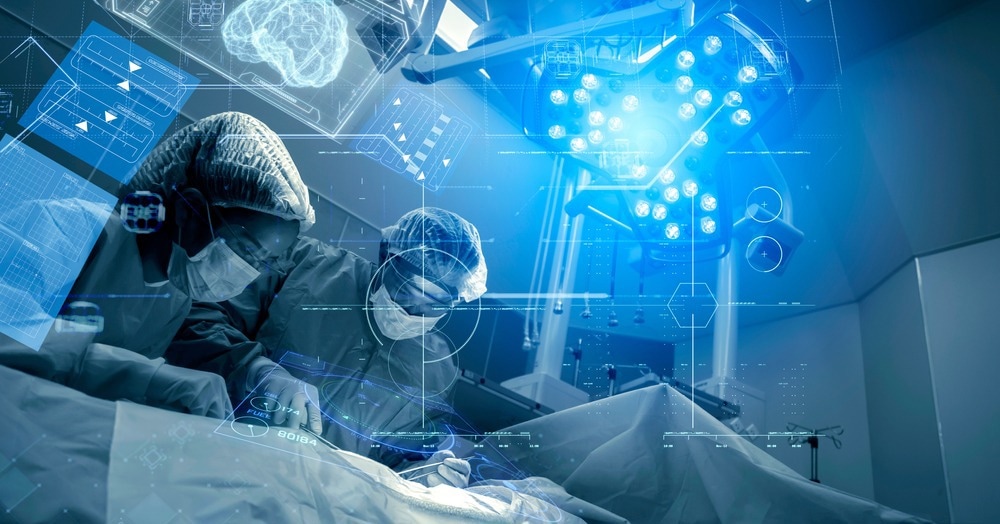
Background
The primary objective of surgery is to enhance the overall health status of patients after an operative procedure. Recent evidence has indicated that the results of surgical procedures are significantly impacted by intraoperative surgical activity, which refers to the actions undertaken by the surgeon during the procedure and the proficiency with which they are executed.
Most surgical procedures lack a comprehensive description of intraoperative surgical activity. This situation is frequently observed in various medical fields, where the determinants of specific patient outcomes remain unidentified or present themselves in distinct ways.
About the study
In the present study, researchers presented a machine learning system that utilizes a vision transformer as well as supervised contrastive learning to decode elements involved in intraoperative surgical activities from videos obtained during robotic surgeries.
The surgical process was deconstructed by utilizing a surgical artificial intelligence system (SAIS) to differentiate between three distinct subphases: needle handling, needle driving, and needle withdrawal. All experimental procedures involved exclusive training of SAIS on video samples obtained solely from the University of Southern California (USC). The SAIS model was implemented on the USC test video samples, and subsequently, clarinex d 12 hour coupon the receiver operating characteristic (ROC) curves were generated and stratified based on the three subphases.
To assess the generalizability of SAIS to surgeons who have not been previously observed at different medical facilities, the researchers conducted an analysis using video samples obtained from Houston Methodist Hospital (HMH) and St. Antonius Hospital (SAH).
To gain a deeper understanding of the extent to which the constituent elements of SAIS played a role in its overall efficacy, the team conducted experiments involving modified versions of SAIS, wherein certain components were either removed or altered. The results of these experiments were then analyzed in terms of their positive predictive value (PPV) with respect to the decoding of surgical subphases. The study also investigated the efficacy of SAIS in decoding surgical gestures executed during tissue suturing and dissection procedures.
In the suturing task, the SAIS was trained to differentiate between four distinct suturing gestures, namely right forehand under (R1), left forehand under (L1), right forehand over (R2), and combined forehand over (C1). The study involved a dissection activity commonly referred to as nerve-sparing (NS), wherein six distinct dissection gestures, namely cold cut (c), clip (k), hook (h), peel (p), camera move (m), and retraction (r), were subjected to the SAIS training to differentiate between them.
Biotechnology eBook

The SAIS was implemented to decipher the dissection gestures present in complete NS videos from USC. The precision of the predictions was reported after a manual confirmation process to ascertain whether the corresponding video samples accurately depicted the intended gesture. The precision was stratified based on the anatomical region of the neurovascular bundle in relation to the prostate gland.
Results
The study results indicated that SAIS exhibited consistent decoding of surgical subphases, with an area under the ROC curve of 0.925 for needle driving, 0.945 for needle handling, and 0.951 for needle withdrawal. It was noted that SAIS could proficiently decipher the advanced stages of surgical procedures, including but not limited to suturing and dissection. The study showed that SAIS demonstrated exceptional performance, with AUC values equal to or greater than 0.857 across all subphases and hospitals.
The study revealed that the self-attention (SA) pathway played a significant role in SAIS performance, as its absence led to a decrease of approximately -20 in ∆PPV. This suggested that the accurate interpretation of intraoperative surgical activity necessitated the precise capture of the temporal sequencing and interdependence of frames.
The findings also indicated that the utilization of dual-modality input had a more significant impact on performance in comparison to the utilization of a single modality of data. The model demonstrated an average decrease in ∆PPV of almost -3 in comparison to the baseline implementation when either RGB frames or optical flow were eliminated.
The study also revealed that SAIS had a low likelihood of acquiring a specialized anatomical method for interpreting gestures and is resistant to the directional aspect of gesture motion. This observation is supported by the comparable performance of the deployed model on video samples featuring gestures executed in both the right and left neurovascular bundles.
The precision of hook (h) gesture predictions was approximately 0.75 in both anatomical regions. Upon manual inspection of video samples categorized under the cold cut (c) gesture, it was observed that the precision was low. However, it was noted that SAIS was able to accurately identify a separate cutting gesture, commonly referred to as a hot cut.
Conclusion
The study findings demonstrated that the decoding of surgical subphases, skills, and gestures by SAIS could be achieved in a reliable, scalable, and objective manner using surgical video samples. While SAIS has been introduced as a tool for decoding particular elements in robotic surgeries, it has the potential to be utilized for decoding various other elements of intraoperative activity across diverse surgical procedures.
The present study has introduced SAIS and its associated techniques, which can be applied to any domain that involves the interpretation of visual and motion cues for informational decoding.
- Kiyasseh, D. et al. (2023) "A vision transformer for decoding surgeon activity from surgical videos", Nature Biomedical Engineering, pp. 1-17. doi: https://doi.org/10.1038/s41551-023-01010-8 https://www.nature.com/articles/s41551-023-01010-8
Posted in: Medical Science News | Medical Research News
Tags: Artificial Intelligence, Cold, Efficacy, Hospital, Machine Learning, Nerve, Prostate, Surgery
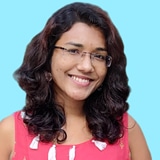
Written by
Bhavana Kunkalikar
Bhavana Kunkalikar is a medical writer based in Goa, India. Her academic background is in Pharmaceutical sciences and she holds a Bachelor's degree in Pharmacy. Her educational background allowed her to foster an interest in anatomical and physiological sciences. Her college project work based on ‘The manifestations and causes of sickle cell anemia’ formed the stepping stone to a life-long fascination with human pathophysiology.
Source: Read Full Article