maxalt spc
Artificial intelligence (AI) models have been validated for recognizing signs of eye diseases in retinal images, enhancing diagnosis, and risk stratification. Combining natural images and medical data, these models provide reliable disease prediction, thereby enabling efficient risk stratification in fields like chest X-rays and dermatology imaging.
In a recent study published in Nature, researchers present retinal image foundation model (RETFound), a self-supervised learning (SSL) masked autoencoder-based foundation model for retinal images. RETFound learns generalizable representations from unlabeled retinal images, which served as the foundation for label-efficient model adaption in various applications.
Study: A foundation model for generalizable disease detection from retinal images. Image Credit: GeebShot / Shutterstock.com
About the study
RETFound is a SSL model that was trained on 1.6 million unlabeled retinal images. An improved SSL-based approach was used on natural images and retinal images retrieved from the Moorfields diabetic image dataset (MEH-MIDAS) with population data to develop two separate models.
MEH-MIDAS is a retrospective dataset containing the full ocular imaging records of 37,401 diabetic patients examined at Moorfields Eye Hospital between January 2000 and March 2022. RETFound was fine-tuned with task labels before verifying its performance on a range of difficult detection and prediction tasks.
Ocular illness diagnostic categorization, prognosis, cytotec oral abortion and oculomic problems including the three-year estimation of cardiovascular disorders like myocardial infarction, cardiac failure, and ischemic stroke, and neurodegenerative disease like Parkinson's disease, were assessed. The illness-detection ability of RETfound was investigated using variable-controlling tests and qualitative findings, whereas its performance and generalizability in adjusting to varied ocular activities after pretraining on retinal scans were examined.
RETFound was tested using diabetic retinopathy databases of MESSIDOR-2, the Indian diabetic retinopathy image (IDRID), and Kaggle APTOS-2019, which were classified according to the International Clinical Diabetic Retinopathy Severity scale. Cross-evaluation was performed between the three databases and models were fine-tuned using a single dataset before being tested on the other ones.
The internal performance of AlzEye data was determined for the one-year prognosis of another eye transitioning to wet macular degeneration. Four oculomic challenges were devised to assess the effectiveness of the model in predicting the occurrence of systemic disorders using retinal scans.
RETFound was trained to detect general structural abnormalities for the diagnosis of systemic disorders.
Study findings
With fewer labeled data, the Adapted RETFound model regularly surpassed many comparator models in the diagnosis and prognosis of sight-threatening eye illnesses, as well as the incident prediction of complicated systemic ailments including heart failure and myocardial infarction. As compared to state-of-the-art rival models, including those pre-trained on ImageNet-21k using classical transfer learning, RETFound consistently outperformed these models in terms of its performance and label efficiency.
The most prominent imaged areas reflected existing information from ocular and oculomic literature. In most datasets, RETFound performed the best, followed by SL-ImageNet.
On the MESSIDOR-2, IDRID, and Kaggle APTOS-2019, datasets, RETFound obtained area under the receiver operating curve (AUROC) values of 0.9, 0.8, and 0.9, respectively, which significantly surpassed SL-ImageNet.
Superior performance was also observed in categorizing several illnesses such as glaucoma. The findings of RETFound AUPR were similarly considerably higher than those of the comparable groups.
In terms of the prognosis of ocular diseases, RETFound outperformed the comparator groups considerably, with an AUROC value of 0.9. RETFound had the greatest AUROC value of 0.8 using color fundus photography (CFP) as the input modality, which was significantly higher as compared to SSL-Retinal. Furthermore, the RETFound AUPR scores were greatest with color fundus photographs and equivalent to the SSL-Retinal model using optical coherence tomography (OCT).
RETFound had an AUROC value of 0.7 for predicting myocardial infarctions using color fundus photographs, whereas SSL-Retinal ranked second but was considerably poorer than RETFound. RETFound also outperformed the other AI models considering OCT images as inputs imaging modality.
RETFound demonstrated improved label efficiency across many tasks, thus highlighting the potential utility of this approach to ease data shortages. Consistently good adaptation efficiency was also observed, which implies that RETFound required less time to adjust to downstream tasks. RETFound discovered and inferred the representation of illness-related regions using SSL for eye disease detection, thereby contributing to performance and label efficiency in downstream operations.
Anatomical structures related to systemic disorders were highlighted as regions contributing to the prediction of incidences of systemic disorders in oculomic tasks. RETFound maintained consistent performance, even when the age difference was reduced, thus demonstrating that this model detected disease-related anatomical structural changes and utilized the data to forecast systemic disorders.
Conclusions
RETFound is a generalizable method for increasing retinal imaging performance and strengthening AI applications' diagnostic and prognostic capabilities. This model employs SSL on unlabeled and natural retinal images, thereby exceeding the strong SL-ImageNet and improving the overall performance of medical foundation models.
- Zhou, Y., Chia, M.A., Wagner, S.K. et al. (2023). A foundation model for generalizable disease detection from retinal images. Nature. doi:10.1038/s41586-023-06555-x. www.nature.com/articles/s41586-023-06555-x
Posted in: Device / Technology News | Medical Science News | Medical Research News | Medical Condition News
Tags: Artificial Intelligence, Dermatology, Diabetic Retinopathy, Diagnostic, Eye, Eye Disease, Glaucoma, Heart, Heart Failure, Hospital, Imaging, Ischemic Stroke, Macular Degeneration, Myocardial Infarction, Neurodegenerative Disease, OCT, Optical Coherence Tomography, Parkinson's Disease, Retinopathy, Stroke, Tomography
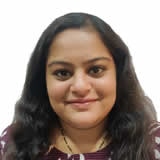
Written by
Pooja Toshniwal Paharia
Dr. based clinical-radiological diagnosis and management of oral lesions and conditions and associated maxillofacial disorders.