Machine learning unlocks secrets of antimicrobial resistance, identifying over 900 key genes
In a recent study published in the journal Nature Communications, researchers investigated the genetic determinants of antimicrobial resistance (AMR). They combined multiple cutting-edge techniques, including gene annotation, pangenomics, and machine learning, to identify AMR genes in 12 species of bacteria commonly exhibiting resistance to 69 currently prevalent drugs. Their analyses revealed over 900 known and novel genes responsible for AMR in these species. They further validated a machine-learning-based workflow outperforming conventional AMR gene identification tools such as Pyseer. Finally, in the BW25113 Escherichia coli strain, they identified conditional resistance and the mechanism underpinning this observation, thereby paving the path for future antibiotics that may solve this alarming global problem.
Study: Global pathogenomic analysis identifies known and candidate genetic antimicrobial resistance determinants in twelve species. Image Credit: Saiful52 / Shutterstock
The burden of AMR and the challenges in combatting it
Antimicrobials are naturally derived or synthetically designed chemicals, including antibiotics, antivirals, antifungals, and antiparasitics, used to prevent and treat infectious diseases in humans, animals, and plants. Antimicrobial Resistance (AMR) occurs when bacteria, viruses, fungi, and parasites no longer respond to antimicrobial medicines, mainly due to the drug-induced selection for variants with genetic compositions that allow them to break the drug down into less harmful substances or escape the drug’s mode of action altogether.
AMR is a globally prevalent and growing concern, with bacteria capable of resisting almost all currently known antibiotics. This resistance is estimated to be directly responsible for more than 700,000 annual deaths, with the number expected to exceed 10 million by 2050 unless substantial interventions are implemented. Recent research has generated tens of thousands of bacterial genome sequences and resistance metadata, which are being used to elucidate the genetic underpinnings of the condition in search of a cure.
Given the significant amount of publically available data, most global analyses of AMR have shifted to a machine-learning (ML) approach for predicting AMR phenotypes using genetic variation datasets. While models have been developed for Escherichia coli, Mycobacterium tuberculosis, Klebsiella pneumoniae, Salmonella enterica, and others, results present a discordance between genetically identified and experimentally established mechanistic determinants of AMR. This suggests that our current knowledge of the field remains lacking and deters the adoption of ML systems for real-world therapeutic interventions.
Statistical approaches using genome-wide association studies (GWAS) have been developed to overcome this demerit. While more accurate in their predictive power, these tools, including PLINK, GEMMA, DBGWAS, and Pyseer, tend to overestimate dataset features. This con notwithstanding, ML depicts a promising tool in the medical researcher’s arsenal against AMR.
About the study
In the present study, researchers develop a novel AMR genetic workflow comprising a combination of systematic gene annotation, pangenome construction, and ML-based support vector machine (SVM) training to elucidate the genes responsible for AMR bacterial phenotypes of 12 prevalent pathogenic bacterial species.
The dataset used in this study was obtained from the PATRIC database and accessed on 21 August 2021. The World Health Organization (WHO) global priority pathogen list (2017) and the ESKAPEE pathogen list were used to select the 12 target pathogens. Inclusion criteria for included GWAS data comprised genome status (complete), the number of contigs in the sequence, total genome length, and the number of drugs each species was resistant to.
The sequence clustering approach was employed for species-specific gene, allele, and open reading frame (ORF)-flanking sequence variant discovery, genetic feature identification, and pangenome construction. Species that depicted at least 100 susceptible intermediate resistant (SIR) phenotypes (SIR) or minimum inhibitor concentrations (MICs) were noted. CARD ontology was used for AMR identification and classification.
Alleles corresponding to identified AMR genes were combined, de-duplicated, and re-clustered for cross-species resistance transmission identification. SVM model training consisted of using each species-drug pair to classify genome sequences as AMR susceptible or non-susceptible based on the species’ AMR-associated features.
“To accelerate training, feature count was reduced in three stages: 1) features present or missing in less than 3 genomes were removed, 2) perfectly correlated features were merged, and 3) remaining features were sorted by log odds ratio (LOR) for resistant genomes.”
SVM model performance was tested using 5-fold cross-validation methodologies. The mean Matthews correlation coefficient (MCC) was used to evaluate the predictive accuracy of SVM models. These results were then compared against Pyseer and Fisher’s exact test predictions for the same dataset to assess the benefits (if any) of the SVM approach over the most commonly used current tests.
Study findings
This study comprised 27,155 individual GWAS samples from 12 species and identified 176,911 SIR phenotypes. Analyses revealed 142 AMR genetic features, two of which were experimentally verified in the BW25113 E. coli strain. Analyses of 6,332 known and novel candidate AMR genes revealed that 14.6% (925) were present in multiple studied species and were mainly vector-bound. This is corroborated by previous work suggesting that gene or feature transfer usually occurs in plasmid-bound genetic material compared to chromosomally encoded material.
Interestingly, while gene/feature commonality was observed in different variants of the same species and closely related species, cross-genera, and cross-class transfer were extremely rare, with only eight genes identified across distantly related genera.
“It has been suggested that transfer of AMR genes between unrelated species such as between gram-positive (GP) and gram-negative (GN) species is rare but possible, having been inferred for tetracycline resistance proteins, ermB, aph(3’), and observed for some beta-lactamases”
The novel SVM ensembles were able to identify and recover almost twice as many AMR genes compared to the commonly used Pyseer and Fisher’s exact test when applied to 127 species-drug cases. Model performance was better than both the latter tests, as was accuracy. When testing a 1000 SIRs dataset where known AMR genes were randomly hidden, the SVM models were able to identify those regions with high GWAS scores. Furthermore, the model was found to require significantly fewer estimators for hyperparameter tuning than previously described models and hence needed much less computational investment than conventional MLs.
a Candidate identification workflow. From each trained AMR-ML model, the top 10 predictive features were identified, filtered for features that are neither known AMR genes nor very rare, ranked based on statistical evidence for resistance in other related drugs, and finally categorized by functional annotation. When multiple features related to the same gene were predictive of resistance, the feature with the strongest evidence was selected and the others were labeled as correlates. Mobile genetic element is abbreviated MGE. b Distribution of 43 well-characterized AMR gene candidates by species and drug class.
Conclusions
The present study used a novel SVM ensemble model to identify, classify, and predict phenotypes of AMR bacteria. It was able to elucidate that one of the mechanisms of the rapid acquisition of resistance in bacterial populations in cross-species plasmid-bound gene transfer. However, this is primarily restricted to closely related species and rarely occurs between distantly related genera. The SVM model verified known AMR genes and discovered novel genes while performing much better than conventional ML and statistical tools despite requiring less computational power.
“Overall, combining pangenomics, systematic gene annotation, and ML provides a workflow for efficiently uncovering patterns of known and candidate AMR genes at the scale of 10,000 s of genomes with potentially greater reliability than contemporary statistical methods such as Pyseer or Fisher’s exact test. Continued development is necessary to bring ML into the current GWAS toolbox when mining sequencing data for previously unknown genetic determinants underlying complex phenotypes.”
- Hyun, J. C., Monk, J. M., Szubin, R., Hefner, Y., & Palsson, B. O. (2023). Global pathogenomic analysis identifies known and candidate genetic antimicrobial resistance determinants in twelve species. Nature Communications, 14(1), 1-17, DOI – https://doi.org/10.1038/s41467-023-43549-9, https://www.nature.com/articles/s41467-023-43549-9
Posted in: Device / Technology News | Medical Science News | Medical Research News | Disease/Infection News
Tags: Allele, Antimicrobial Resistance, Bacteria, Chemicals, Drugs, E. coli, fungi, Gene, Gene Transfer, Genes, Genetic, Genome, Infectious Diseases, Machine Learning, Pathogen, Plasmid, Research, Salmonella, Tetracycline, Tuberculosis
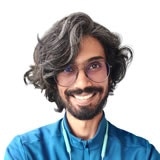
Written by
Hugo Francisco de Souza
Hugo Francisco de Souza is a scientific writer based in Bangalore, Karnataka, India. His academic passions lie in biogeography, evolutionary biology, and herpetology. He is currently pursuing his Ph.D. from the Centre for Ecological Sciences, Indian Institute of Science, where he studies the origins, dispersal, and speciation of wetland-associated snakes. Hugo has received, amongst others, the DST-INSPIRE fellowship for his doctoral research and the Gold Medal from Pondicherry University for academic excellence during his Masters. His research has been published in high-impact peer-reviewed journals, including PLOS Neglected Tropical Diseases and Systematic Biology. When not working or writing, Hugo can be found consuming copious amounts of anime and manga, composing and making music with his bass guitar, shredding trails on his MTB, playing video games (he prefers the term ‘gaming’), or tinkering with all things tech.